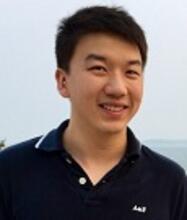
The question answered in this thesis is whether it is possible to construct a rigorous and efficient perturbation method to obtain the effective static Hamiltonian of a nonlinear oscillator driven by a time-dependent oscillatory force. We present in this dissertation such a method, based on Feynman-like diagram. The pictorial structure associated with each diagram corresponds directly to a Hamiltonian term, the prefactor of which involves a simple counting of topologically equivalent diagrams. We also leverage the algorithmic simplicity of our scheme in a readily available computer program that generates the effective Hamiltonian to arbitrary order. At the heart of our diagrammatic method is a novel canonical perturbation expansion developed in phase space to capture the quantum nonlinear dynamics. A merit of this expansion is that it reduces to classical harmonic balance in the limit of ħ -> 0. Our method establishes the foundation of the dynamic control of quantum systems with the precision needed for future quantum machines. We demonstrate its value by treating five examples from the field of superconducting circuits. These examples involve an experimental proposal for the Hamiltonian stabilization of a three-legged Schrödinger cat, modeling of energy renormalization phenomena in superconducting circuits experiments, a comprehensive characterization of multiphoton resonances in a driven transmon, a proposal for an inductively shunted transmon circuit, and a characterization of classical ultra-subharmonic bifurcation in driven oscillators. Lastly, we benchmark the performance of our method by comparing it with experimental data and exact Floquet numerical diagonalization.
Thesis advisor: Michel Devoret (michel.devoret@yale.edu)