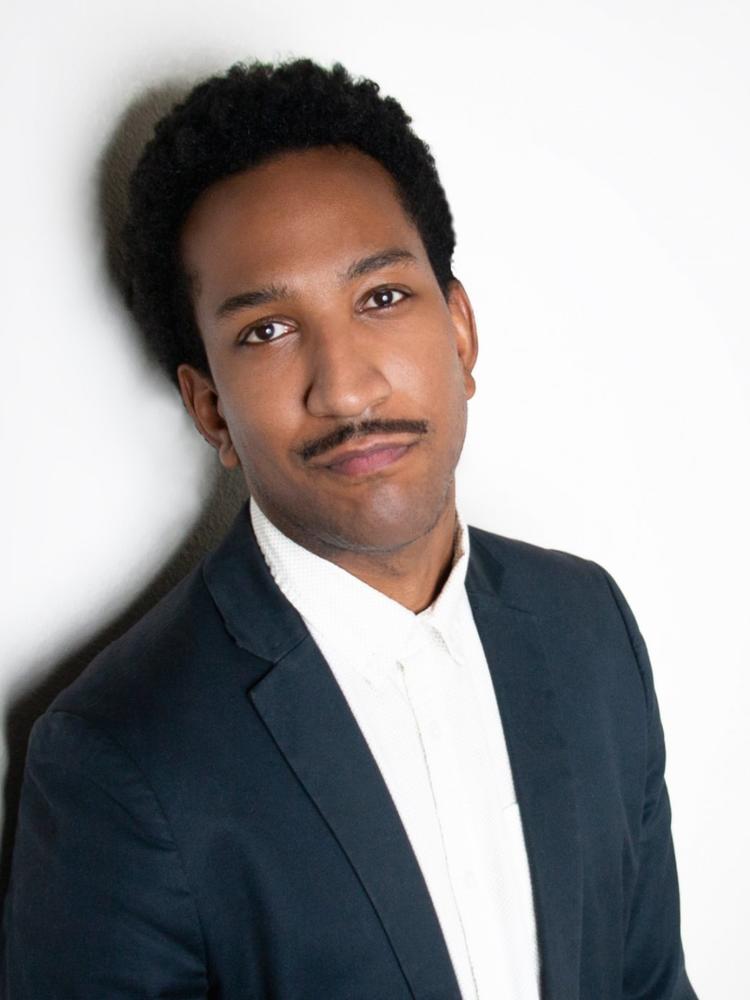
“Model-free inference from time-series data”
Drawing inferences from experimental data often involves imposing models, which can lead to inaccurate conclusions. Theory sometimes points to quantities that are significant independent of the underlying mechanisms, but making model-free estimates of these quantities is hard because finite data generates systematic errors. I present two cases where we develop new methods to address and correct these errors: (1) extracting long-term population growth from single-cell lineage data and (2) estimating the evidence for the arrow of time in patterns of neural activity. For population growth, key observables are division counts and generation times. Simple growth rate estimators suffer from finite-time bias at short times; this scales inversely with time and can be corrected. At longer times, rare events introduce a linearization bias, causing an abrupt transition understood by mapping to the Random Energy Model. Accurate estimates occur when lineage counts and lengths stay below the critical point, allowing inference of how mutations and physiological variations impact fitness. In the case of neural activity, the relevant observables are the moments of activity and the waiting times between them. Estimating the evidence for irreversibility—the Kullback-Leibler divergence between the distribution of forward and reverse trajectories—faces similar biases. Finding the systematic dependence of these biases on sample size allows accurate estimates, including detecting systems that obey detailed balance, and opens a path to exploring how the brain represents the arrow of time. Generally, this new understanding of how model-free estimators rely on a complex order of limits of the amount of data and the length of each sample may allow quantitative understanding of other relevant processes such as gene regulation, cell-cycle dynamics, and other coarse-grained systems.
Host: Mark Gonzalez, Joseph Lap, Xinping Yang