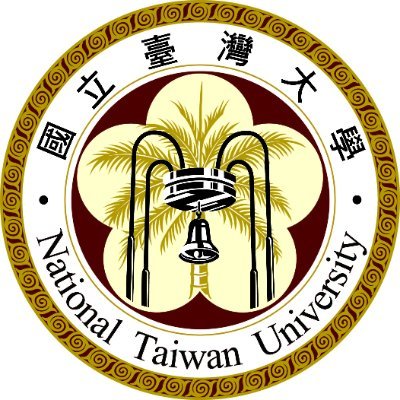
“Truncatable Conformal Bootstrap with Reinforcement Learning”
We present a reinforcement-learning framework to identify spectra that can potentially originates from a truncatable physical theory. In the work, we derive a statistical quantity that is related to the error of cluster decomposition between abandoned heavy states and the vacuum, and we interpreted this as truncability. By focusing on two dimensional conformal field theory, we apply reinforcement learning techniques to minimize such quantity, and single out candidate spectra that have the origin of minimal models such as Ising model and Lee-Yang singularity.
Also, we demonstrate that the neural network indeed learns to find “minimization” algorithm through an example of transfer learning. At the same time, this shows that our technique is applicable to find both unitary and non-unitary theories.
Host: David Poland