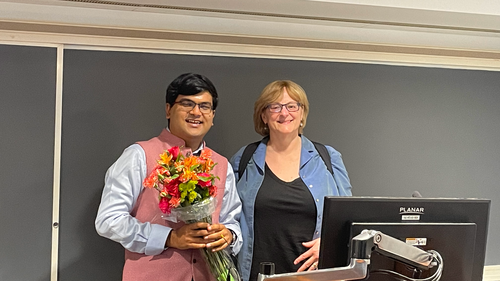
On Monday, July 31, Aritra Ghosh, an astronomy student, successfully defended the thesis, “Investigating Galaxy Morphology in Large Surveys Using Novel Machine Learning Frameworks” (advisor: Meg Urry).
Aritra stated, “The shapes and sizes of galaxies have played an instrumental role in our understanding of galaxy formation and evolution. For my thesis, I worked on developing new machine-learning frameworks that can accurately determine the shapes and sizes of billion-galaxy samples expected over the next decade in astrophysics. We used these frameworks to create one of the largest galaxy shape/size catalogs currently available – containing ~8 million galaxies from the Hyper Suprime-Cam Survey. This large sample has helped us to understand how the sizes of galaxies change as a function of their environment – a topic that has seen a lot of conflicting results. Using our tools, we have conclusively established that galaxies in denser environments are 10-20% larger than their equally massive counterparts in less dense regions of the Universe. This result is now helping us shed new light on the intricate relationship between galaxies and the dark matter halos that they reside in.”
Aritra has been been awarded the 4-year LSSTC Catalyst Fellowship funded by the John Templeton Foundation. He will be starting his fellowship at the DiRAC Institute at the University of Washington. He will be continuing his work on developing novel techniques to harness large data volumes and shed new light on the physics of galaxy formation and evolution.
Thesis abstract
The morphological features and structural parameters of galaxies serve as a cornerstone in comprehending their attributes and have played an instrumental role in our understanding of galaxy formation and evolution. In this work, we develop novel machine learning frameworks that can determine the morphology of billion-galaxy samples expected over the next decade. We demonstrate that our frameworks can be applied across a wide variety of ground and space-based datasets, can be trained with minuscule amounts of real data, and can predict ~30-60% better uncertainties than existing morphology-determination tools.
We use our frameworks to create one of the largest structural parameter catalogs currently available, containing ~8 million Hyper Suprime-Cam galaxies. This catalog represents a significant improvement in size (~10X), depth (~4 mag), and uncertainty quantification over current state-of-the-art bulge+disk decomposition catalogs.
We use this large sample to present one of the first comprehensive studies of the variation of galaxy radius with the environment – a relationship that has remained enigmatic with over a decade of conflicting results. With >5\sigma confidence, we confirm that galaxies in denser environments are ~10-20% larger than equally massive counterparts in less dense regions of the Universe. We verify the presence of the above correlation separately for disk-dominated, bulge-dominated, star-forming, and quiescent sub-populations. We posit that the above correlation could be driven by assembly bias in dark matter halos and the varying prevalence of galaxy mergers in different environments.