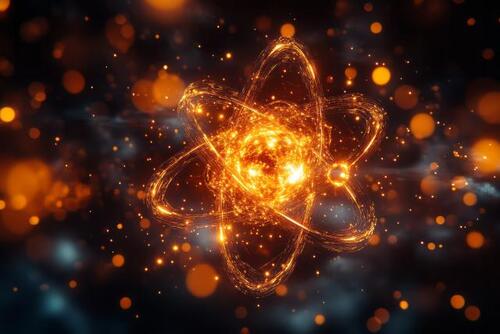
Figuring out certain aspects of a material’s electron structure can take a lot out of a computer - up to a million CPU hours, in fact. A team of Yale researchers, led by Diana Qiu, Assistant Professor of Mechanical Engineering and Physics, are using a type of artificial intelligence to make these calculations much faster and more accurately. Among other benefits, this makes it much easier to discover new materials. The results are published in Nature Communications.
In the field of materials science, exploring the electronic structure of real materials is of particular interest, since it allows for better understanding of the physics of larger and more complex systems, such as moiré systems and defect states. Researchers typically will use a method known as density functional theory (DFT) to explore electronic structure, and for the most part it works fine.
“But the issue is that if you’re looking at excited state properties, like how materials behave when they interact with light or when they conduct electricity, then DFT really isn’t sufficient to understand the properties of the material,” said Qiu.
In that case, she said, researchers turn to higher-level theories that build on top of DFT. The problem for Qiu, though, is that her research focuses on problems that tend to be very computationally expensive. Sometimes, researchers will solve for this by applying machine learning to their calculations. But it’s not much help in figuring out a material’s band structure, Qiu said, “which is what’s necessary to really understand the properties of the material.”
Qiu and her team decided to focus on the electrons’ wave function, which mathematically describes the quantum state of a particle. For the study, they used 2D materials, which are just a few atoms in thickness.
“We said ‘Okay, what is the wave function?’” said Qiu, assistant professor of mechanical engineering & materials science. “It’s this probability over space. We can always plot this as an image in space.”
They used a variational autoencoder, an AI image-processing tool to create a dimensional representation of the wave function.
“This is done in what’s called an unsupervised way, so there’s no human guidance,” she said. “It takes this 100-gigabyte object and reduces it to 30 numbers, and those numbers represent the initial wave function. We then use that as the input for a second neural network that can then predict these more complicated excited state properties.”
Because the method avoids relying on intuition-based human guidance, it provides a more accurate representation. It’s also much more efficient and can be generalized for other applications.
“The representation that we can end up getting is also about 100 to 1,000 times smaller than what you need for feature selection,” she said. That’s important because the simpler the input, the better it can be used for other applications.
Qiu notes that conventional methods of calculating the band structure of something with three atoms could take between 100,000 and a million CPU hours. Their VAE-assisted method takes about an hour.
“The immediate practical application is that it gives us a way of really speeding up these complicated calculations, which means that now we can do these calculations for a wider range of materials, and that enables the discovery of new materials with properties that we care about.”
This story was adapted from the Yale School of Engineering and Applied Sciences news article of December 18, 2024. Please see below for a link to the original article and to the referenced article in Nature Communications.