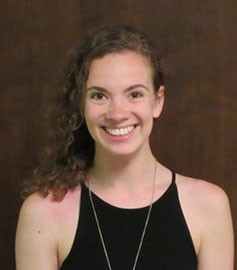
On March 9, 2021, Mariel Pettee successfully defended the thesis: “Interdisciplinary Machine Learning Methods for Particle Physics” (Advisor: Sarah Demers).
Pettee explained, “my thesis describes machine learning (ML) methods I’ve created to solve very different problems across high-energy particle physics, with a focus on the ATLAS Experiment at CERN. First, I discuss using neural networks designed for sequential data to improve our identification of true hadronic tau decays in the ATLAS detector during live datataking runs, meaning the decision must be made in a split second using a reduced amount of information than what is available offline. Next, I show results from the application of Variational Autoencoders (VAEs) and Graph Neural Networks (GNNs) to generate and analyze 3D motion capture data of myself as an example of how to apply generic ML techniques to many-body particle systems. These methods have applications to urgent problems in high-energy physics such as track reconstruction, event simulation, and anomaly detection. Finally, I describe the search for the Higgs boson decaying to a tau pair and produced in association with a leptonically-decaying vector boson with the full Run 2 ATLAS dataset. This is one of the few major Higgs boson interactions we might access with the LHC that, as of yet, has not been observed. Thanks to a new ML-based background estimation technique I’ve introduced, as well as a sophisticated estimation of our misidentified backgrounds, we have a strong handle on the many sources of background events for this low-statistics Higgs boson measurement.”
Pettee will join Berkeley Lab as a Chamberlain Fellow, developing new machine learning models for applications in high-energy physics and other areas of fundamental science.
Thesis Abstract: Following the discovery of a Higgs boson-like particle in the summer of 2012 at the Large Hadron Collider (LHC) at CERN, the high-energy particle physics community has prioritized its thorough study. As part of a comprehensive plan to investigate the many combinations of production and decay of the Standard Model Higgs boson, this thesis describes a continued search for this particle produced in association with a leptonically-decaying vector boson (i.e. a W or Z boson) and decaying into a pair of tau leptons.
In Run 1 at the LHC, ATLAS researchers were able to set an upper constraint on the signal strength of this process at μ = σ/σ_SM < 5.6 with 95% confidence using 20.3 fb^-1 of collision data collected at a center-of-mass energy of √s = 8 TeV. My thesis work, which builds upon and extends the Run 1 analysis structure, takes advantage of an increased center-of-mass energy in Run 2 of the LHC of √s = 13 TeV as well as 139 fb^-1 of data, approximately seven times the amount used for the Run 1 analysis. While the higher center-of-mass energy in Run 2 yields a higher expected cross-section for this process, the analysis faces the additional challenges of two newly-considered final states, a higher number of simultaneous interactions per event, and a novel neural network-based background estimation technique. I also describe advanced machine learning techniques I have developed to support tau identification in the ATLAS High-Level Trigger as well as predicting and analyzing the dynamics of many-body systems such as 3D motion capture data of choreography.