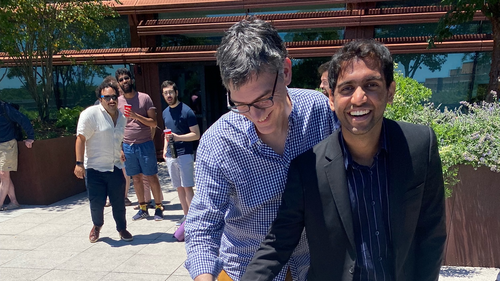
On June 28, Viraaj Jayaram successfully defended the thesis “Normative and Empirical Investigation of the Role of Odor Timing in Drosophila Olfactory Navigation” (advisor: Thierry Emonet).
Viraaj explained, “Across the animal kingdom, animals must navigate odor signals to their sources, in order to find food and mates. This is often a challenging task as turbulent flow effects can make odor signals highly spatiotemporally complex. In my thesis, I use computational and theoretical approaches to find good strategies for solving this problem and analyze experimental data taken from navigating fruit flies to better understand the strategies they employ. This work helps us contextualize and make future predictions for animal navigation behavior and neural information processing.”
He will be working as a Machine Learning Engineer at Schrödinger, in NYC, where he will help build and maintain physics-based software for drug design and materials science.
Thesis abstract:
Many organisms must navigate attractive odor signals to find food or mates. However, this is not a simple task; odor signals can be spatiotemporally complex and depend on the flow conditions and terrain. In this talk, I present computational studies that suggest normative strategies for this task, alongside an analysis of olfactory navigation decisions in walking Drosophila. Our simulations show that detecting two complementary temporal features of odor signals supports robust navigation across diverse environments. Furthermore, we find that models of the Drosophila olfactory circuit are sensitive to two features in a manner useful for navigation. Additionally, analysis of real behavioral data indicates that flies do use these features in navigation, as predicted. Finally, I present ongoing research employing deep reinforcement learning to optimize the integration of various directional cues into a goal heading for navigating complex odor plumes. These insights yield testable hypotheses regarding the navigation behavior of flies and other organisms.