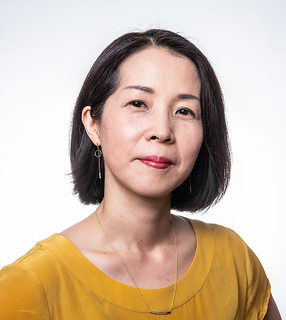
With the exciting opportunities brought by advancements in quantum hardware technologies, it is crucial to develop systematic methods to reliably and efficiently extract information from limited quantum state measurements. We propose an attention-based neural network called quantum attention networks (QuAN), which is tailored for quantum state measurement data by respecting the permutation invariance of individual snapshots and attending to high-order correlations between different snapshots. The power of the QuAN is demonstrated through three examples:
(1) QuAN successfully learns entanglement transition from hard-core boson experiments, only using the Z-basis measurements.
(2) QuAN learns the increasing complexity of deep random circuits from random circuit experiments.
(3) QuAN saturates the error threshold in learning the topological state in the noise-controlled simulated surface code data.
The examples establish QuAN as a general-purpose machine for learning quantum complexity from limited mixed-state data. I will discuss what the results imply for a versatile decoding scheme.