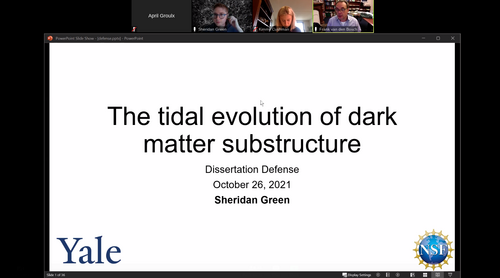
On October 26, 2021, Sheridan Green successfully defended the thesis “The tidal evolution of dark matter substructure and the significance of halo-to-halo assembly history variance”. (Advisor: Frank van den Bosch).
Green states “Dark matter subhaloes currently offer one of our best opportunities to constrain the nature of dark matter. The primary way that we make predictions of subhalo statistics is via cosmological N-body simulations. These simulations have been an incredible tool for science, but they suffer from limitations due to their discrete nature. During my time at Yale, I helped develop SatGen (led by Fangzhou Jiang), which is a modeling framework that is complementary to simulations that allows us to make independent predictions of subhalo statistics. We used this model to demonstrate that cosmological simulations underpredict the abundance of subhaloes by ~10-20%, which is attributed to the artificial destruction of subhaloes that arises due to runaway instabilities triggered by discreteness noise. This result has important implications for constraints placed on the particle properties of dark matter made by indirect detection experiments. We also used SatGen to help explain that the cause of the tension between the simulated abundance of subhaloes near the halo center and observed abundance of satellite galaxies near the halo center arises due to the finite mass resolution of the simulations.”
On his future plans “I’m heading to work in trading, where I’ll be joining Susquehanna International Group as a Quantitative Strategist in a couple of weeks.”
Thesis Abstract: Accurately predicting the abundance and structural evolution of dark matter subhaloes is crucial for understanding galaxy formation, large-scale structure, and constraining the nature of dark matter. Due to the nonlinear nature of subhalo evolution, cosmological N-body simulations remain its primary method of investigation. Subhaloes in such simulations have recently been shown to still be heavily impacted by artificial disruption, diminishing the information content (at small scales) of the simulations and all derivative semi-analytical models calibrated against them.